
Daniela Calvetti
James Wood Williamson Professor, Department of Mathematics, Applied Mathematics, and Statistics
Case Western University
2:00 p.m. E.D.T. | Fri., May 20, 2022 | 1324 East Hall
Better Together: The Partnership of Bayesian Inference and Numerical Analysis in the Solution of Inverse Problems
The numerical solution of inverse problems where the number of unknowns exceeds the available data is a notoriously difficult problem. Regularization methods designed to over come the paucity of data penalize candidate solutions for unlikely or undesirable features. Discretization level of the underlying continuous problem can also be used to improve the accuracy of the computed solution. In this talk we show how recasting the inverse problems within the Bayesian framework makes it possible to express via a probability density function features believed to characterize the solution in a way that interfaces naturally with state of the art computational schemes. In that context, we will present an efficient computational scheme for the recovery of sparse solutions, where the sparsity is encoded in terms of hierar chical models whose parameters can be set to account for the sensitivity of the data to the solution. The computations can be organized as an inner-outer iteration scheme, where a weighted linear least squares problem is solved in the inner iteration and the outer iteration updates the scaling weights. When the least squares problems are solved approximately by the Conjugate Gradient method for least squares (CGLS) equipped with a suitable stopping rule, typically the number of CGLS iterations quickly converges to the cardinality of the support, thus providing an automatic model reduction. Computed examples will illustrate the performance of the approach in a number of applications.
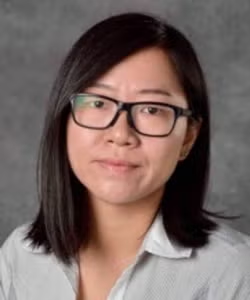
Yingda Chen
Professor, Department of Mathematics and Department of Computational Mathematics, Science and Engineering
Michigan State University
3:00 p.m. E.D.T. | Fri., May 20, 2022 | 1324 East Hall
Sparse Grid Discontinuous Galerkin (DG) Methods for High Dimensional PDEs
The numerical solution of inverse problems where the number of unknowns exceeds the available data is a notoriously difficult problem. Regularization methods designed to over come the paucity of data penalize candidate solutions for unlikely or undesirable features. Discretization level of the underlying continuous problem can also be used to improve the accuracy of the computed solution. In this talk we show how recasting the inverse problems within the Bayesian framework makes it possible to express via a probability density function features believed to characterize the solution in a way that interfaces naturally with state of the art computational schemes. In that context, we will present an efficient computational scheme for the recovery of sparse solutions, where the sparsity is encoded in terms of hierar chical models whose parameters can be set to account for the sensitivity of the data to the solution. The computations can be organized as an inner-outer iteration scheme, where a weighted linear least squares problem is solved in the inner iteration and the outer iteration updates the scaling weights. When the least squares problems are solved approximately by the Conjugate Gradient method for least squares (CGLS) equipped with a suitable stopping rule, typically the number of CGLS iterations quickly converges to the cardinality of the support, thus providing an automatic model reduction. Computed examples will illustrate the performance of the approach in a number of applications.

Lothar Reichel
Professor, Department of Mathematical Sciences
Kent State University
9:00 a.m. E.D.T. | Sat., May 21, 2022 | 1324 East Hall
Error Estimates for Golub-Kahan Bidiagonalization with Tikhonov Regularization for Ill-posed Operator Equations
Linear ill-posed operator equations arise in various areas of science and engineering. The presence of errors in the operator and the data often makes the computation of an accurate approximate solution difficult. We compute an approximate solution of an ill-posed operator equation by first determining an approximation of the operators of generally fairly small dimension by carrying out a few steps of a continuous version of the Golub-Kahan bidiag onalization (GKB) process to the noisy operator. Then Tikhonov regularization is applied to the low-dimensional problem so obtained and the regularization parameter is determined by solving a low-dimensional nonlinear equation. The effect of replacing the original oper ator by the low-dimensional operator obtained by the GKB process on the accuracy of the solution is analyzed, as is the effect of errors in the operator and data. Computed examples that illustrate the theory are presented. This talk presents joint work with A. Alqahtani, T. Mach, and R. Ramlau.

Peijun Li
Professor, Mathematics
Purdue
10:00 a.m. E.D.T. | Sat., May 21, 2022 | 1324 East Hall
Inverse Random Source Problems for Wave Equations
Motivated by significant applications, the inverse source problem remains an important and active research subject in inverse scattering theory. The inverse random source problem refers to the inverse source problem that involves uncertainties, and is substantially more challenging than its deterministic counterpart.
In this talk, our recent progress will be discussed on inverse source problems for the stochastic wave equations. I will present a new model for the random source, which is as sumed to be a microlocally isotropic Gaussian random field such that its covariance operator is a classical pseudo-differential operator. The well-posedness and regularity of the solution will be addressed for the direct problem. For the inverse problem, it is shown that the prin cipal symbol of the covariance operator can be uniquely determined by the high frequency limit of the wave field at a single realization. I will also highlight some ongoing and future projects in the inverse random potential and medium problems.

Zhimin Zhang
Professor, College of Liberal Arts and Sciences
Wayne State University
2:00 p.m. E.D.T. | Sat., May 21, 2022 | 1324 East Hall
Efficient Spectral Methods and Error Analysis for Nonlinear Hamiltonian Systems
We investigate efficient numerical methods for nonlinear Hamiltonian systems. Three polynomial spectral methods (including spectral Galerkin, Petrov-Galerkin, and collocation methods) are presented. Our main results include the energy and symplectic structure pre serving properties and error estimates. We prove that the spectral Petrov-Galerkin method preserves the energy exactly and both the spectral Gauss collocation and spectral Galerkin methods are energy conserving up to spectral accuracy. While it is well known that collo cation at Gauss points preserves symplectic structure, we prove that the Petrov-Galerkin method preserves the symplectic structure up to a Gauss quadrature error and the spectral Galerkin method preserves the symplectic structure to spectral accuracy. Furthermore, we prove that all three methods converge exponentially (with respect to the polynomial de gree) under sufficient regularity assumption. All these aforementioned properties make our methods possible to simulate the long time behavior of the Hamiltonian system. Numerical experiments indicate that our algorithms are efficient.

Li Wang
Assistant Professor, School of Mathematics
University of Minnesota
3:00 p.m. E.D.T. | Sat., May 21, 2022 | 1324 East Hall
Variational Computational Methods for Gradient Flow
In this talk, I will introduce a general variational framework for nonlinear evolution equations with a gradient flow structure, which arise in material science, animal swarms, chemotaxis, and deep learning, among many others. Building upon this framework, we develop numerical methods that have built-in properties such as positivity preserving and entropy decreasing, and resolve stability issues due to the strong nonlinearity. Two specific applications will be discussed. One is the Wasserstein gradient flow, where the major chal lenge is to compute the Wasserstein distance and resulting optimization problem. I will show techniques to overcome these difficulties. The other is to simulate crystal surface evolution, which suffers from significant stiffness and therefore prevents simulation with traditional methods on fine spatial grids. On the contrary, our method resolves this issue and is proved to converge at a rate independent of the grid size.